Conversations with Ken Wong
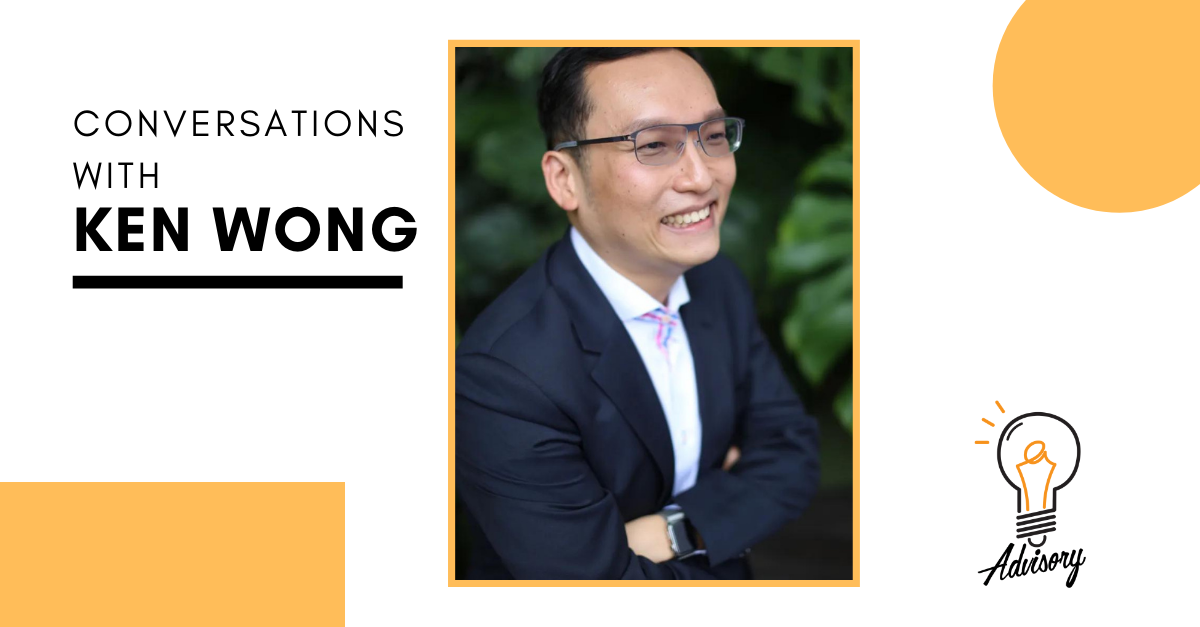
By Jolie Fong and Adriale Pang
Mr. Ken Wong is currently the Head of Data Science, Asia Pacific at Visa. Ken has more than 20 years of experience in the fields of data analytics, analytical marketing, CRM, customer intelligence, database and event-based marketing. Previously, he founded the AI Lab at OCBC to develop the consumer bank’s expertise in artificial intelligence. He has worked with financial, telecommunication, technology and fast-moving consumer goods (FMCG) organisations in the region to help create strategic business values by unlocking their data assets through analytical techniques. He has also taken on regional consulting and business development roles within business consulting and marketing services organisations.
Outside of his professional role, Mr. Wong has been an invited guest speaker at various universities, workshops and conferences where he shared his expertise on database marketing and analytics. He has also published various data mining research papers at leading conferences around the world.
Tell us about yourself and what you do.
I am the Head of Data Science for Visa in Asia Pacific. Visa is the leading global digital payments network and we facilitate secure and reliable transactions between consumers, merchants, banks and governments. My role is to help Visa’s clients serve their customers better by leveraging the huge amount of transaction data Visa gathers when payment is made on a Visa credential. We help card issuers understand how their customers transact and what kinds of products and services might make the experience better.
Other than working with card issuers or banks, we work with internal stakeholders to help them gain insight in terms of finding new business opportunities and operational efficiency.
To do all this, we work very closely with clients to understand their pain points and use cases, and we have to constantly challenge ourselves to innovate and build new techniques and algorithms that bring new perspectives.
I have been in the domain of data science and analytics for more than 20 years. Prior to Visa, I was with OCBC and founded the OCBC AI Lab. I have also taken on regional consulting roles with companies like IBM.
What does your typical workday look like?
On a typical day, I could be doing several things. One would be checking in with my team and making sure projects are on track. I could also be meeting with key clients in the region and understanding their business pain points and advising them on data strategies. I might be speaking with internal stakeholders to find out how data science can be used to help them advance their businesses. I also find myself working with data and technology partners to collaborate and share potential data use cases. Or I could be talking to budding data scientists to understand if a career at Visa would fit their aspiration. I wouldn’t say there is a single day in my week that is the same.
What are some of the projects you have worked on, whether for Visa’s external clients or internal stakeholders?
For card issuers or banks, their objective is to serve customers better. One of the studies we do is to look at a card issuer’s customer base and examine how Visa cards are used after activation. Many times, customers start with a small transaction, before moving into daily expenditures like at the supermarket or petrol station. Sooner or later, they make bigger purchases, like a smartphone. We study these patterns within the issuer’s base, compare that against other card issuers, and provide recommendations on what can be done to better incentivise customers of different profiles to be more active on their cards.
For our internal stakeholders, a recent project was with the company’s economics team, where we studied how COVID-19 has been impacting spending behaviours and looking for signs of when and where recovery might happen. For example, if consumers think the economy is reopening or feel more financially secure, they tend to spend more in certain categories. We monitor these trends over time and use the insights to inform the company’s business decisions.
How do you differentiate between artificial intelligence, data science, and data analytics?
The basic skills are probably similar. The only differentiation would be that data analytics involves more visualisation and storytelling to make recommendations to solve business problems. Data science would be more technical, in terms of building and implementing models that automate decision-making, and is a bit closer to software engineering in some ways. I would say that a budding data scientist would have to be good at both aspects.
People associate the term “artificial intelligence” with all sorts of things. The reality is that AI or data science is nothing but math and statistics looking for patterns across large amounts of data. In recent years, AI has become closer to the things you might see in Hollywood movies, but that is just because many of these AI techniques now understand text documents, images and speech which were traditionally handled by humans. This makes AI look as smart as a human.
What is the culture like in a team of data scientists, especially as these teams grow bigger? How do you avoid a stifling hierarchy?
I run a team of 80 data scientists today, and they are based in different countries and territories, which makes it doubly challenging.
What I like to do is run my team with a start-up like culture, where everyone has a say and voices their opinion, and foster communications and collaborations. You may be a junior data scientist but you have the opportunity to work on a large project, because you have the skills and desire to do so. You’re not restricted just because of your rank or because you’re less experienced.
We also encourage ideation by having lunch-and-learn and brainstorming sessions, and these are still essential parts of how we run data science teams as having different perspectives is critical. We also allow people time off to do research and encourage our data scientists to publish patents and innovate.
What are some trends or challenges your teams have identified and are working on?
I think there are many views on what the future of fintech is like, and I do not want to add to that confusion. But regardless of how the future of fintech turns out, one thing that is certain is the increasing importance of data. The application of data science will continue to be the key differentiating factor.
As an industry, there are a couple of challenges we are working on. One is the constant challenge to create new technology that can process larger and larger data sets.
Finding ways to nurture and attract talent is also a challenge. Even though the industry is still growing, I think companies need to look outside of the traditional talent pool, so that people who do not have that research/technical/math background are still given an opportunity for a career in this field. Given how important ideation is to data science, it is increasingly becoming more crucial to build teams where members hail from a wide range of industries and can offer diverse perspectives.
What was your experience with higher education like? How did it play a part in your career?
I did my undergraduate and postgraduate studies in NUS. My bachelor’s and master’s were in computer science, but my master’s had a focus on data analytics. When I was in university 20 years ago, we didn’t even have the term “data science” – at the time, we called it “data mining”.
My interest in data mining was piqued when one of my professors also shared the classic beer-and-diaper story, which is about how U.S. researchers found that young fathers who go to the supermarket on a Friday evening will pick up both diapers and beer at the same time since they wouldn’t have time to visit pubs. This led some supermarkets to display beer and diapers together, and sales boomed.
That was my introduction to what data mining was. I thought that using data points to investigate trends was fascinating, and that it was going to change how we do things in the future. Nowadays, ‘data mining’ has come a long way; beyond studying correlations, we can now use it for things like fraud detection, recommendations, and training machine algorithms.
Which did you pick first - your higher education major, or your career interest?
I had a passion for data, and I was also doing well in data and math in school, so I naturally flowed into it. You may think it was lucky or unlucky for me, but this was the only domain I delved into across my career.
On the other hand, I know friends who made major transitions in their careers, for example thinking that they were going to go deep into law but switching over to finance instead. There is no one correct template for a career path. When you are in your 20s, you can still explore, but perhaps once you hit your 30s, you could specialise more and start building your portfolio. Then when you are beyond your 40s, you settle down in a particular occupation.
Of all the industries you could have applied analytics to, what was it that led you to fintech and payments in particular?
In the earlier part of my career, I was in consulting, so I got to work with many industries, be it telecommunications, retail or FMCG. We can apply data science to virtually any industry that generates lots of data and has the need to optimise their business. I was on my career journey during a time where financial institutions were going through a transformation, one which data played a key role in, so for me, it was pure coincidence that I landed in banking and fintech.
Do you see a dichotomy between your managerial role versus the more technical data science aspect?
Personally, I enjoy working on data and building algorithms myself, but nowadays I find it even more rewarding to build talented teams and motivate them to collaborate and work towards a common vision.
I still want to get my hands dirty occasionally, but managing teams is often unavoidable as we advance in our careers. At some point we must ask ourselves: do we want to go deeper into technical excellence, or have a broader career? I chose to focus more on building my team.
Are there any misconceptions about your field that you would like to clear up?
People have this misconception that all data scientists are predominantly left-brained – good in logical thinking, lateral thinking, math and sequencing. But I would argue that a good data scientist is also right-brained, possessing thinking skills related to visualisation, intuition, and holistic thinking.
I have met many data scientists who are great musicians and artists, and I like photography a lot. So you see us engaging in right-brained functions too, and it may be reductive to pigeonhole us into being strictly left-brained.
What skill sets would a good hire possess? If you were to hire someone, which would be the priority - having a background in finance/economics, or data science?
A good data scientist needs to have a multidisciplinary outlook, which comprises:
- A good understanding of math and data
- A good coder
- Have good storytelling skills – being able to translate data into insights and share them with stakeholders in a useful format.
A hacker mindset is also important – always having that curiosity, always wanting to drill deeper and deeper into a particular problem, and never being satisfied. This is seldom talked about in schools as learning technical skills like coding often take priority over such soft skills.
I have worked with great data scientists who never thought they would come into data science – some were from hospitality, others from aerospace engineering. It is true that they have an elementary proficiency in coding, but ultimately what was more important was their hacker mindset. Increasingly our tools can help us abstract away the math and technical skills required, and instead what cannot be taught is that creative problem-solving mindset.
What can students do to discover more about, and orient themselves to, a career in this field?
One great thing about data science is the huge open-source community. There is a lot of content online related to data science, which helps to close the knowledge gap. Students can easily gain access to sample data sets and ideas. For example, if you want to build an AI to recognise song titles, you could find an open-source project online and learn about it over the weekend. You may not be able to master it, but slowly you will build up your skills.
Start with the product you want to create for a real-life use case, then work your way back to learn the skills needed to create it. It may not be very helpful to your data science career if you start from the other way round, i.e., learning math, differentiation, and integration, before thinking about their potential applications in the real world.
There’s a lot to learn, and students tend to focus on the technical aspect, learning Python for example. But I think students should complement their efforts by exploring a particular functional domain. Be it finance, images, or sounds, identify an area you are interested in then explore how you can apply data science to it.
All great innovations do not just start with engineering. Sure, the Nespresso machine has great engineering behind it, be it the building of the capsules or high-pressure pumps, but there is that human aspect too, knowing coffee, the taste, the water, and the powder. You need to develop some knowledge in a particular functional domain, for example knowledge of coffee in the food and beverage industry, then apply your engineering skills to it.
Looking back, is there anything you wish you had known when you first started working?
Back then when I first started, this industry did not exist. It was mainly just a research domain, known mainly as “data mining”. There was no “data science”, nor “data analytics”. There was a lot of doubt in my mind whether this would be a viable career path. But maybe many of my doubts were unnecessary.
I had asked myself whether I wanted to be a software engineer, and my answer was no. Did I want to be part of an exciting, up-and-coming industry? Yes. Did I see data having a future? Yes. So I stuck to my gut, and everything turned out well, so I am lucky. I think if I had hindsight, I need not be so worried.
Any bite-sized piece of advice for youths thinking of pursuing a career in data science?
It might be tough at the start, but don’t give up. Tinker a lot. You can always start exploring as a hobby and build up a sufficiently good portfolio. Do not be discouraged if you do not have a PhD in this domain. Academic credentials may be helpful, but many employers including myself are increasingly looking at real world application and experience, and the intuition and creative mind as more important skills to have.